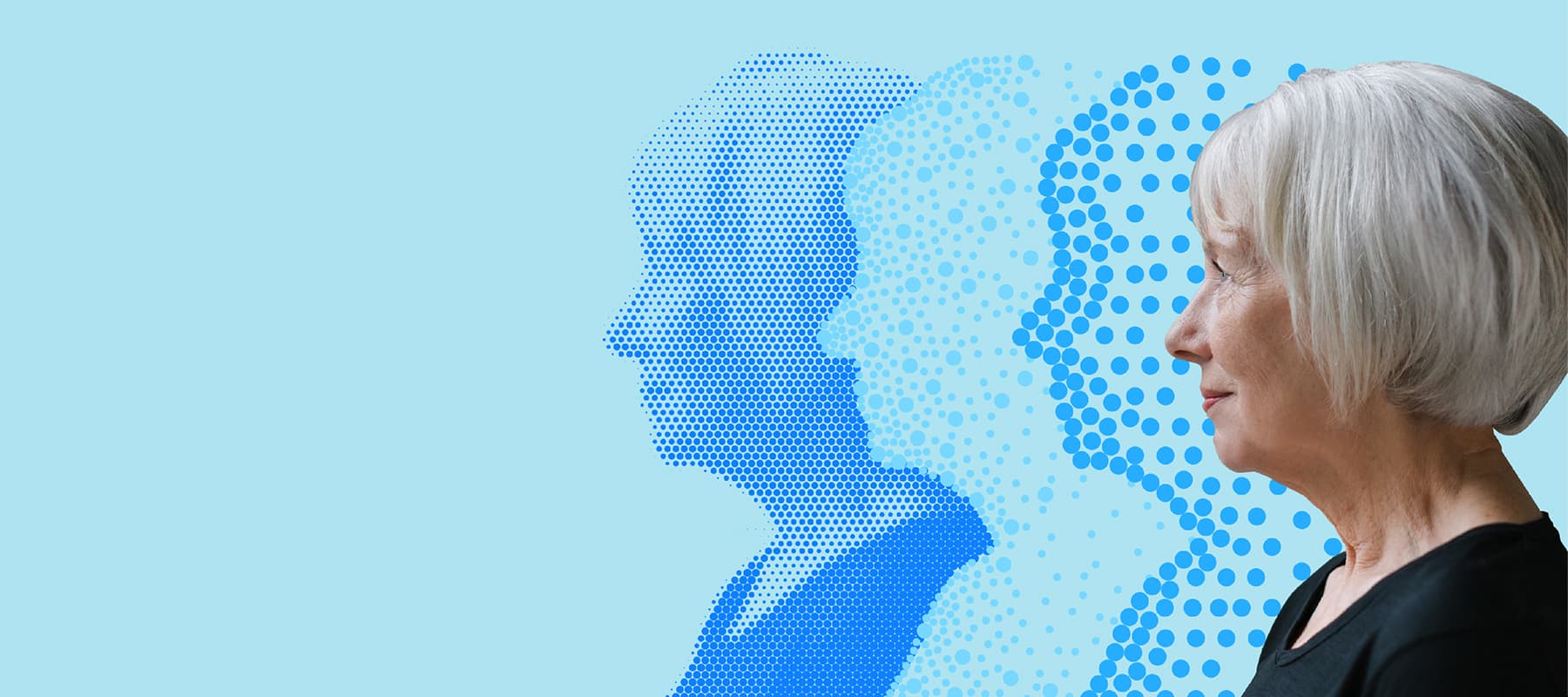
Incorporating External Data into Clinical Trials: Comparing Digital Twins to External Control Arms
Most drug development professionals are familiar with the nerve-racking wait for the read-out of a large trial. If it’s negative, is the investigational therapy ineffective? Or could the failure result from an unforeseen flaw in the design or execution of the protocol, rather than a lack of efficacy? The team could spend weeks analyzing data, but a definitive answer may be elusive due to insufficient power for such analyses in the already completed trial. These problems are only made worse if the trial had lower enrollment, or higher dropout than expected due to an unanticipated event like COVID-19. And if a trial is negative, the next one is likely to be larger and more costly — if it happens at all.
- The pharmaceutical industry spends over $80 billion on R&D each year.1
- It takes 12 to 15 years on average for an experimental drug to travel from the lab to U.S. patients.2
- A phase III clinical trial can require up to 3,000 patient volunteers and only approximately 25% to 30% of drugs move to the next phase.3
Sponsors frequently use external control arms to shorten timelines and cut costs for proof-of-concept studies. Unfortunately, this opens the door to confounders — differences between the patients in the external control arm and those in the treatment arm that make it impossible to attribute differences in outcomes to the effect of the treatment. As a result, trials with external control arms are efficient but unreliable.
The availability of large historical datasets of longitudinal patient information and the rapid development of artificial intelligence (AI) technologies mean that clinical trials don’t have to remain stuck in this status quo. It’s possible to have the best of both worlds: the reliability of a randomized controlled trial coupled with the efficiency of an external control arm. The innovation that makes this possible is called a Digital Twin.
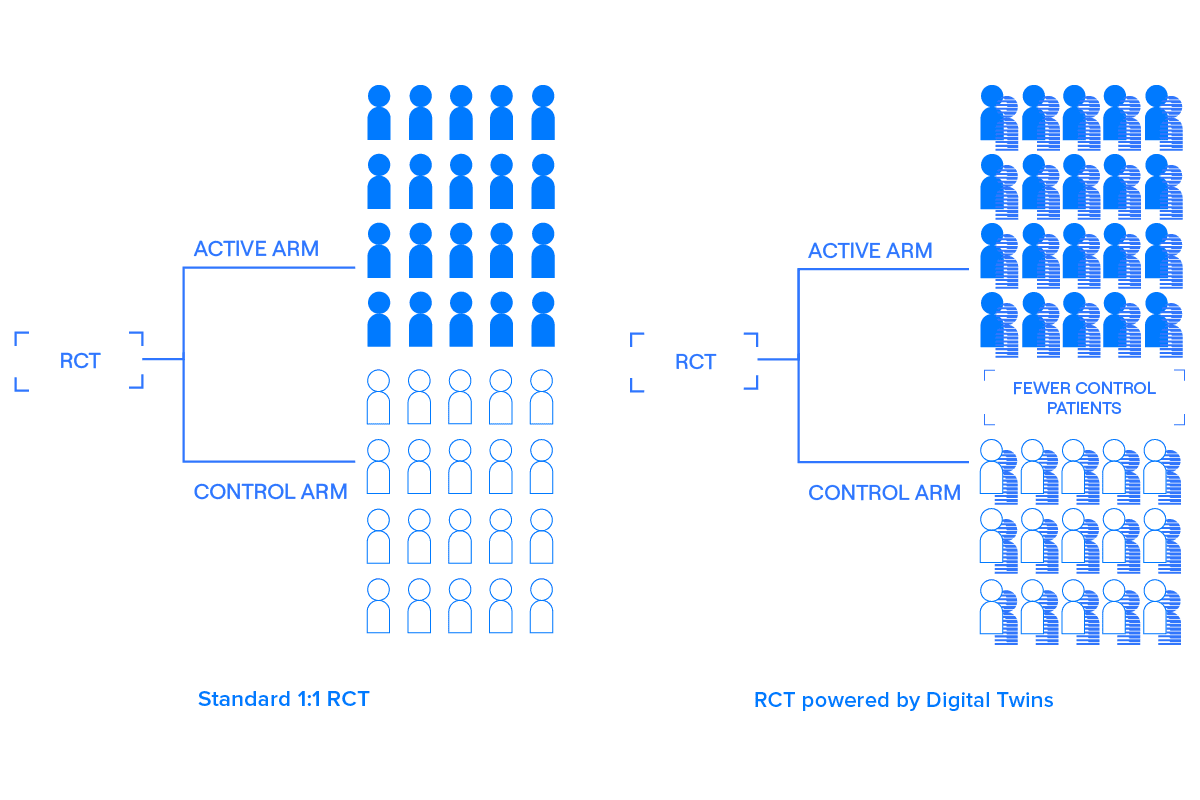
A Digital Twin is a comprehensive, longitudinal clinical record created using the baseline data collected from a patient — before they receive their first treatment — that predicts how that patient would likely evolve over the course of the trial if they were to be given a placebo. That is, a Digital Twin is like a simulated control group for a particular patient.
Digital Twins are treated as covariates optimized to explain variability in the outcome. By using pre-specified covariate adjustment — effectively, comparing predicted placebo outcomes to actual placebo outcomes and correcting any bias — Digital Twins can be incorporated into randomized controlled trials to improve power and efficiency without introducing bias.4
Digital Twins are not Synthetic Controls. Synthetic Control Arms (SCAs) add patients who were not in the original patient sample of the trial. Because SCAs can increase Type I error, use cases are limited based on regulatory guidance.5 Digital Twins add information about patients already in the trial. Because these predicted outcomes are treated as covariates, they do not introduce bias, and can be applied to all stages of clinical development.
Read Unlearn.AI’s whitepaper, Incorporating External Control Arms into Clinical Trials, to learn about SCAs and Digital Twins, their respective statistical techniques, and effects on trial operating characteristics. Although both approaches increase statistical power, unlike SCAs, Digital Twins are robust to known and unknown founders.
References
1https://www.cbo.gov/publication/57025
2https://www.fdareview.org/issues/the-drug-development-and-approval-process/
3https://www.fda.gov/patients/drug-development-process/step-3-clinical-research#Clinical_Research_Phase_Studies
4https://arxiv.org/abs/2012.09935
5https://www.fda.gov/regulatory-information/search-fda-guidance-documents/e10-choice-control-group-and-related-issues-clinical-trials