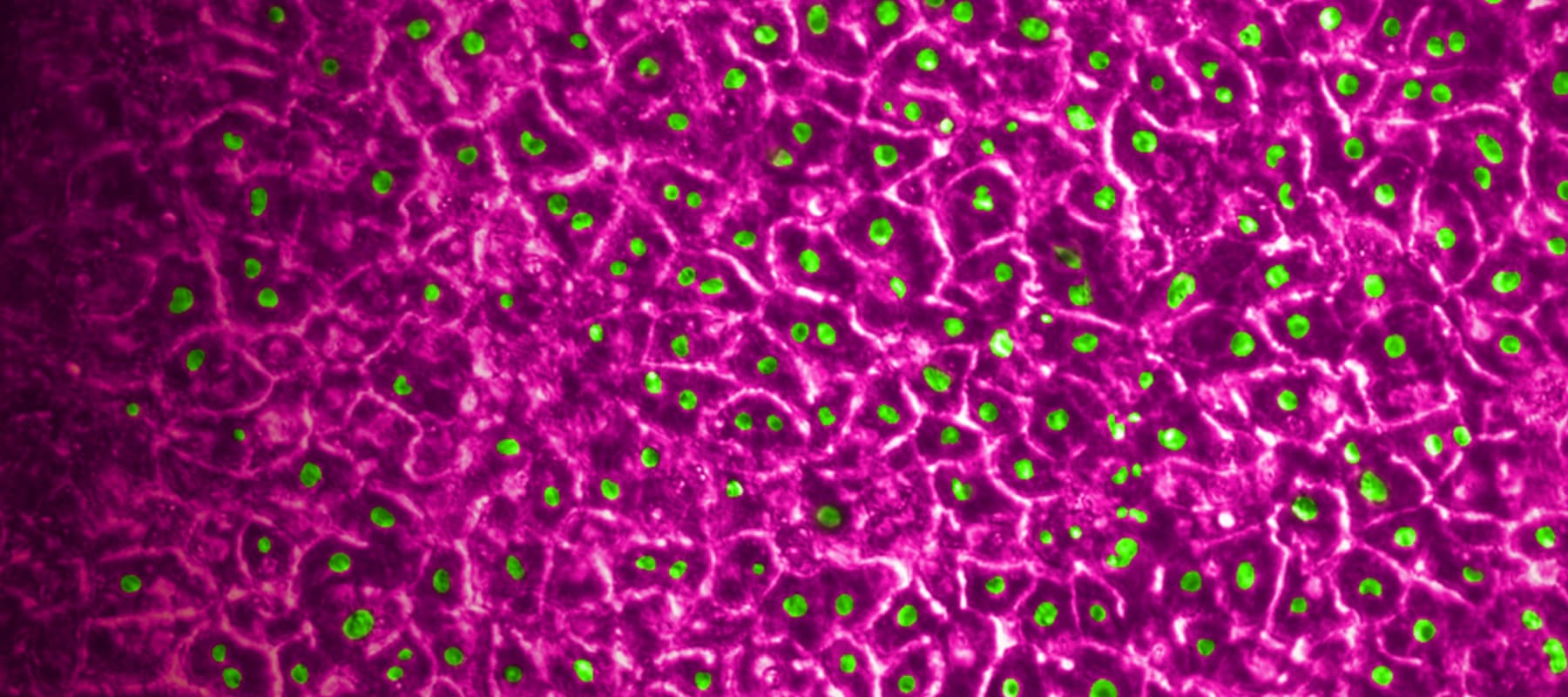
No Good Drug Left Behind: Sensitivity and Specificity in Drug Development Explained
Drug-induced liver injury (DILI), a leading cause of safety-related clinical trial failure and market withdrawals around the world, has been a persistent threat to drug development for decades1,2. Animals like rats, dogs, and monkeys serve as the last line of defense against DILI, catching the toxic effects that drugs could have before they reach humans. Yet, differences between species severely limit these models, and the consequences of this gap are borne out in halted clinical trials and even patient deaths.
Put simply, non-human preclinical models limit the number of life-saving drugs that make it to market—and have dire consequences if they are wrong.
In preclinical drug development, researchers use a wide range of models—including animals, spheroids, and transwells—to determine which drugs should advance to clinical trials. Whether or not scientists make the right decision depends largely on the quality of the models they use. And that quality is measured as both sensitivity and specificity.
The Importance of Model Sensitivity
In this context, “sensitivity” describes how often a model successfully identifies a toxic drug candidate as such. So, a model with 100% sensitivity would correctly flag all harmful drug candidates. If the model system allows a toxic drug to pass through without a strong indication of harm, it will support a false conclusion that the drug is non-toxic—a result colloquially known as a “false-negative.”
False-negatives can enable harmful drug candidates to reach human trials. To avoid this, researchers have long sought models that closely approximate the human body. For more than 80 years, animals have filled that need; however, they are far from perfect. Genetic and physiological differences can produce discrepancies in drug response—a drug that appears safe in rats may turn out to be lethal in humans. Such a result would be described as a false-negative.
As roughly 90% of drugs that enter clinical trials fail—many due to safety concerns—it is clear that animal models are far from 100% sensitive3. Unfortunately, researchers have yet to produce robust data on animal models’ sensitivity, particularly with respect to preclinical toxicology4. Perhaps the main reason why is their assumption that animals are as good as it gets—a belief that can eclipse their desire for proof. However, given the drug failure rate—30–40% of which is due to toxicity responses—animals presumably did not provide sufficient evidence to forecast drug toxicity in humans, and using more sensitive models could have helped researchers in predicting these drugs’ toxic effects.
Researchers measure a model’s sensitivity by screening a set of test drug candidates. It is essential that the set of drugs be carefully selected. Otherwise, one could, for example, bias the test drugs towards “easy” drugs that are very toxic in ways that even simple models would identify; however, showing that a new model can do this likely does not demonstrate its utility in capturing the difficult drugs that are deemed safe by animal testing. This would be similar to claiming a telescope’s ability to spot the sun makes it sensitive to observing stars—while technically true, this is irrelevant to real-world challenges. That said, it is not uncommon for preclinical models to simply be tested using highly toxic drugs that never made it to clinical trials5,6.
While sensitivity is important, it is not enough—models must also identify drugs as toxic or non-toxic correctly. That is, they must also have high specificity.
The Give and Take of Specificity and Sensitivity
“Specificity” refers to how accurate a model is in identifying non-toxic drug candidates. A 100%-specific model would never claim that a non-toxic candidate is toxic. Importantly, a model can be 100% sensitive without being very specific. For example, an overeager model that calls most candidates “toxic” may capture all toxic candidates (100% sensitivity) but also mislabel many non-toxic candidates as toxic (mediocre specificity).
Researchers want the most sensitive preclinical toxicology models possible, as higher sensitivity means more successful clinical trials, safer patients, and better economics. However, this cannot come at the cost of low specificity and potentially failing good drugs. An overly sensitive model with a low toxicity threshold would catch all toxic drugs, but it may also misidentify drugs that are actually safe and effective in humans. Good drugs are rare, and considerable effort and investment goes into their development. Even one drug that never reaches the clinic can cost pharmaceutical companies billions and leave a patient population without treatment. Models should do their utmost to classify non-toxic compounds as such—that is, to have 100% specificity.
But how can drug development insist on perfect specificity when no model is perfect? Fortunately, there is a give-and-take between sensitivity and specificity that model developers can take advantage of: One can be traded for the other.
In decision analysis, sensitivity and specificity can be “dialed in” for the model in question. In most cases, this involves setting a threshold when analyzing the model’s output. In a recent study published in Communications Medicine, part of Nature Portfolio, Ewart et al. set a threshold of 375 on the quantitative output of the Emulate human Liver-Chip—an advanced, three-dimensional culture system that mimics human liver tissue; in the case of hepatic spheroids, an older model system, researchers have set a threshold of 50. In both cases, the higher the thresholds, the more sensitive and less specific the model tends to be. These thresholds were selected precisely to dial the systems into 100% specificity.
Ewart et al. found that even while maintaining such a strict specificity, the Liver-Chip achieved a staggering 87% sensitivity. This means that, on top of correctly identifying most of the toxic drugs, the Liver-Chip never misidentified a non-toxic drug in the study as toxic. For drug developers, this means that no good drugs—nor the considerable resources poured into their development—would be wasted. Using models like Organ-Chips that achieve high sensitivity alongside perfect specificity would allow drug developers to deprioritize potentially dangerous drugs without sacrificing good drugs. In all, this could lead to more productive drug development pipelines, safer drugs progressing to clinical trials, and more patient lives saved.
References:
- Craveiro, Nuno Sales, et al. “Drug Withdrawal due to Safety: A Review of the Data Supporting Withdrawal Decision.” Current Drug Safety, vol. 15, no. 1, 3 Feb. 2020, pp. 4–12, https://doi.org/10.2174/1574886314666191004092520.
- Research, Center for Drug Evaluation and. “Drug-Induced Liver Injury: Premarketing Clinical Evaluation.” U.S. Food and Drug Administration, 17 Oct. 2019, www.fda.gov/regulatory-information/search-fda-guidance-documents/drug-induced-liver-injury-premarketing-clinical-evaluation.
- David B. “Factors Associated with Clinical Trials That Fail and Opportunities for Improving the Likelihood of Success: A Review.” Contemporary Clinical Trials Communications, vol. 11, Sept. 2018, pp. 156–164, www.ncbi.nlm.nih.gov/pmc/articles/PMC6092479/, https://doi.org/10.1016/j.conctc.2018.08.001.
- Bailey, Jarrod, et al. “An Analysis of the Use of Animal Models in Predicting Human Toxicology and Drug Safety.” Alternatives to Laboratory Animals, vol. 42, no. 3, June 2014, pp. 181–199, https://doi.org/10.1177/026119291404200306.
- Zhou, Yitian, et al. “Comprehensive Evaluation of Organotypic and Microphysiological Liver Models for Prediction of Drug-Induced Liver Injury.” Frontiers in Pharmacology, vol. 10, 24 Sept. 2019, https://doi.org/10.3389/fphar.2019.01093. Accessed 22 Nov. 2020.
- Bircsak, Kristin M., et al. “A 3D Microfluidic Liver Model for High Throughput Compound Toxicity Screening in the OrganoPlate®.” Toxicology, vol. 450, Feb. 2021, p. 152667, https://doi.org/10.1016/j.tox.2020.152667.