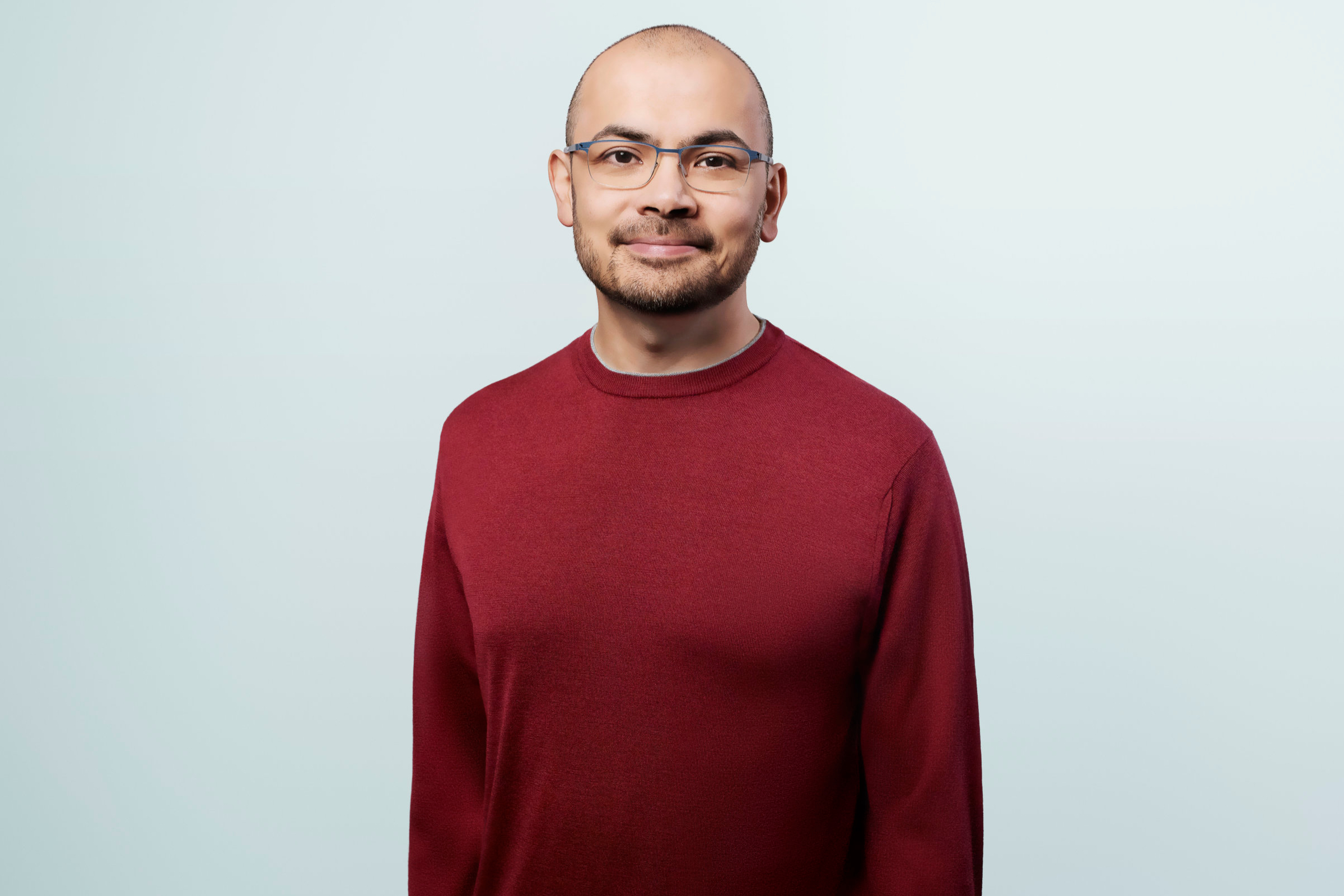
Exclusive: Alphabet's Isomorphic inks first pharma deals with Eli Lilly and Novartis for $83M upfront, $2.9B in milestones
Isomorphic Labs, Alphabet’s closely watched AI startup, has signed its first pharma partnerships, and will work with Eli Lilly and Novartis in deals that could be worth $3 billion, CEO Demis Hassabis exclusively told Endpoints News.
Since its founding in November 2021, Isomorphic has quickly become a leading figure in the fast-growing AI biotech space. The Alphabet subsidiary is applying Google DeepMind’s biology research — particularly its protein-structure predicting model AlphaFold — to drug discovery. Hassabis is CEO of Google DeepMind, where he oversees Alphabet’s AI efforts, as well as CEO of Isomorphic, which now employs about 90 people across its London headquarters and an office in Lausanne, Switzerland.
These first pharma deals, announced the day before the JP Morgan Healthcare Conference gets underway in San Francisco, are Isomorphic’s “lighthouse partnerships,” Hassabis said. Lilly will pay $45 million upfront, along with $1.7 billion in potential milestone payments, to work on several targets. Novartis paid $37.5 million upfront with $1.2 billion in milestones to work on three targets. While the companies are not disclosing targets, both collaborations aim to discover small molecules against hard-to-drug targets.
“They’re not pilot projects. They’re real drug targets,” Hassabis said in an interview. “We want to do things that ultimately, if successful, are really meaningful, actually cure diseases and lead to blockbuster drugs, rather than just pilots and sort of academic work.”
Isomorphic will try virtual screens, molecule design on challenging targets
Isomorphic has had preliminary talks with a range of large drugmakers, Hassabis said, but Lilly and Novartis were the most enthusiastic about getting involved at “ground zero.” Daniel Skovronsky, Eli Lilly’s chief scientific and medical officer, and Fiona Marshall, Novartis’ president of biomedical research, were involved in the deals.
Marshall first heard about AlphaFold when DeepMind’s team won a 2018 protein-folding competition. As Isomorphic got started, Marshall met with Hassabis and Isomorphic’s chief scientific officer Miles Congreve, Marshall’s former colleague at Sosei Heptares. She called the partnership a “natural fit,” as Novartis is also working with other technology giants like Palantir on data storage and Microsoft on generative chemistry.
Marshall asked the disease area teams at Novartis what targets they were struggling with to try out Isomorphic’s ideas.
“These are not easy targets that we’ve picked at all,” she said in an interview with Endpoints. She said they are looking at proteins where the structure isn’t yet solved or chemists can’t find the right type of chemical matter.
Isomorphic will work to find molecules that hit those targets by virtually screening through compounds using high-performance computing. Marshall said a conventional screen typically can cover 1 million compounds, while a virtual screen can consider 20 billion. A second approach that Isomorphic plans to explore is ab initio design, or designing an ideal molecule from scratch to fit into a protein’s pocket.
Hassabis called the two methods — virtual screens and ab initio design — the “obvious low-hanging fruit” for its technology, with promising initial results.
“The proof will be in the pudding, which is why I think we’re ready to take on real drug projects,” he said.
Isomorphic has also progressed AlphaFold to do more than predict static protein structures. Protein structures, by themselves, are “not that helpful for drug design,” Marshall said, and there’s much more value in predicting how a small molecule binds to a particular protein. The next generation of AlphaFold can predict these complex structures. Hassabis called it the “best co-folding system in the world, both for placement of where ligands bind and predicting binding affinity.”
A holy grail for the field is linking the structure of these molecules to function. Marshall called that “the next level to go to” for the technology.
“I don’t think we’re actually there yet,” Marshall said. “All you’re measuring at the moment is just the binding affinity. That doesn’t really tell you what it’s doing to the function of the protein.”
Isomorphic is also working on other models, still under wraps, that could help predict certain properties in compounds like pharmacokinetics and potential toxicity.
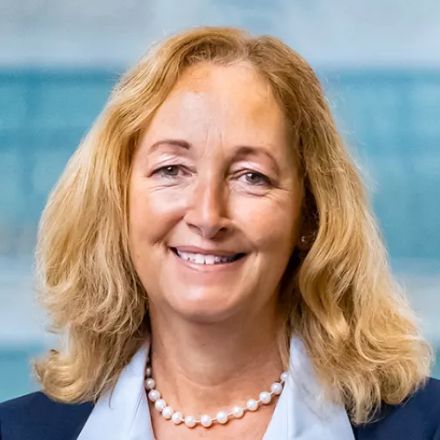
“The low-hanging fruit, which we definitely think Isomorphic is going to be able to do, is reduce the time from when you start with a target to get your candidate molecule,” Marshall said in an interview. “On average, that’s three or four years using conventional approaches. I think it’s reasonable to think you could halve that amount of time.”
Overall, Marshall said she believes AI will help with several, but not all, of the steps in the lengthy process of drug discovery.
“It isn’t going to work miracles,” she said. “Maybe it can make 10 years into six years. I don’t think it’s going to make 10 years into two years.”
General vs. specific models for drug design
The top goal for these collaborations is obvious: making drugs. But Isomorphic has a secondary aim in mind.
Hassabis said he’s interested in learning just how much fine-tuning its models will require to work against specific drug targets. He sees a sliding scale for these models, ranging from the most generalizable systems to the most specific. The Novartis and Lilly partnerships will test just how much a general model can achieve, and where it requires feeding in and training models on specialized data for a certain drug target.
“I don’t know what the optimal balance is between building the general system and doing specialized systems around the specific data or the specific program,” he said. “At the moment, I’m imagining it’s 50-50, but we’ll soon find out. Ideally, I’d like it to be 80-20 towards the general, because that’s more scalable for us as a company.”
Hassabis said he hopes to answer those questions with these first partnerships, before striking more deals.
“For now we’re good, but we’d always be open to discussions and new partnerships, especially if they pushed things in an interesting new way we didn’t already have covered,” he said.
Beyond these collaborations, Hassabis said his aims in 2024 are for Isomorphic’s machine-learning team to keep improving the accuracy of its predictive systems, while the company also progresses its internal pipeline. Details about its own drug programs remain under wraps, but Hassabis said the biotech has shortened a list of about 20 targets down to a handful it’s now going after. The company is using contract research organizations to make and test potential compounds.
“We’re still at the early stages of testing out the predictions of the platform,” Hassabis said. “How reliable are they? Once we’re feeling good about that, then we can really go to town in pushing an internal project really hard.”