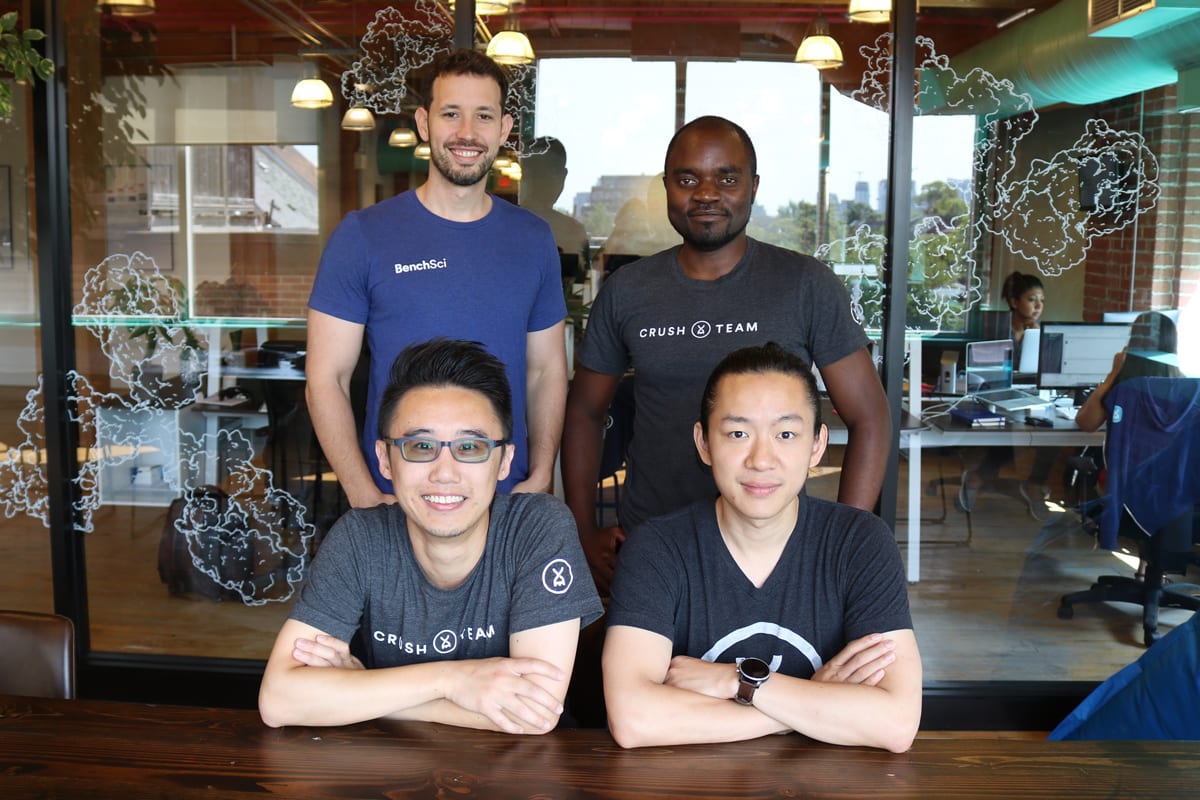
BenchSci founders, clockwise from top left: Liran Belenzon, Elvis Wianda, David Chen and Tom Leung
F-Prime backs a niche AI software startup hunting lofty goals in $22M Series B
For many of the AI companies sprouting on the biopharma field, validation — often meaning confirmation of whether the targets and drugs they identified or generated …
Sign up to read this article for free.
Get free access to a limited number of articles, plus choose newsletters to get straight to your inbox.