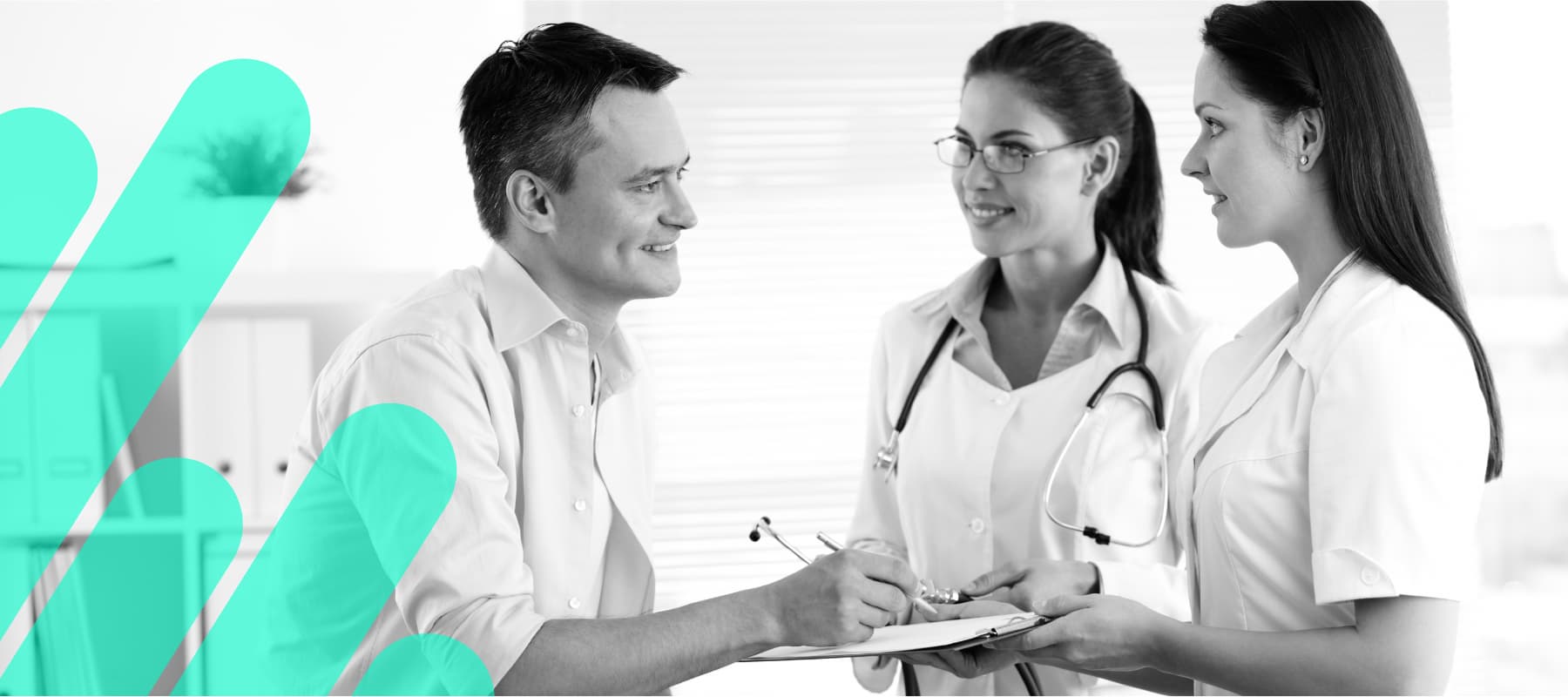
A novel study insight analytics (SIA) methodology improves PANSS data quality and signal detection in a global clinical trial in schizophrenia
Drug development in neuroscience is an extraordinarily challenging process. Many late-stage CNS trials fail, even when testing previously proven effective drugs due to high placebo response and poor signal-to-noise ratios. This results in the cancellation of promising development programs. Such failures represent a tremendous loss of commercial and scientific investment. Robust, practical, proactive methods are badly needed to help improve the likelihood of trial success.
Various quality assurance methods have been proposed and implemented over the last few decades, including WCG’s Study Insight Analytics (SIA) service. SIA uses predefined algorithms to monitor all aspects of data health throughout the conduct of a clinical trial and detect atypical data patterns within and across endpoints. While algorithms have been studied and documented in scientific literature, the actual impact of this method on study outcome has not been well investigated. In our recent publication*, we presented an example of the application of SIA in a Phase III global schizophrenia trial where prospective, real-time evaluation of data quality was conducted; we describe the implementation of detection, analysis, and intervention techniques during the blinded phase of the study; and we review the results of our post-hoc analysis on unblinded data as they apply to the primary study outcome. Additionally, we show how these types of data signals relate to placebo response and signal detection at the group level, and can significantly contribute to clinical trial success.
IDENTIFICATION OF ATYPICAL DATA
The dataset used for the analysis was taken from a phase 3 global clinical study which assessed the efficacy and safety of HP-3070, an asenapine transdermal system (patch), in adults with acutely exacerbated schizophrenia. Fifty-nine study sites from the United States, Russia, Ukraine, Bulgaria and Serbia participated in the study. During the course of the trial, a blinded data analytics program was implemented using electronic data capture and evaluated on a daily basis to identify anomalies in data. Algorithms including data quality markers proposed by the International Society for CNS Clinical Trials and Methodology (ISCTM) as well as periodical review of other in-study performance indicators such as numbers of raters per patient and primary and secondary (PANSS – CGI-S) endpoint correlations were implemented to monitor anomalous data patterns.
INTERPRETATION & INTERVENTION
Any assessments flagged by these algorithms and other quality indicators for data abnormalities resulted in queries to site raters, along with consensus discussions and retraining. The level of intervention was determined by clinical scientists who interpreted the severity of anomalous data. Any rater who failed to improve in response to retraining was asked to discontinue rating. If overall site performance was deemed unsatisfactory and did not demonstrate improvement after early intervention, the site was not permitted to screen additional subjects, thus curtailing their overall contribution to the trial. Implementation of actionable intervention strategy is as important as selecting appropriate algorithms to maintain the integrity of data.
IMPACT
Our post-hoc analysis on unblinded data revealed that greater placebo response was observed for a) sites with moderate to low PANSS – CGI correlation (r < 0.80), b) suboptimal sites with at least one assessment triggering two or more algorithms, c) regions/countries with higher flag rates. In addition, there was a greater association between rater inconsistency and erratic visit-to-visit score changes in the PANSS. These results suggest that quality markers including higher rates of rater inconsistency in PANSS assessments, higher rates of flags within PANSS assessments suggesting low internal concordance, and divergence between PANSS total and CGI-S ratings are indicative of greater placebo response. Addressing these concerns with real-time oversight and rapid intervention to prevent further occurrence of measurement errors would improve signal detection.
DISCUSSION
Findings from this study emphasize the importance of holistically evaluating and remediating each clinical study site as an interventional unit. While individual raters do have a strong impact on the quality of data produced, other factors such as regional differences, resource constraints, and higher rater turnover also contribute. It is noteworthy that while identification of anomalous data can be automated by programing algorithms, it is imperative that clinical experts evaluate the nature and severity of the potential data issues and select the appropriate level of intervention. Each study site plays a crucial role in communicating with the study subjects during trials and they may encounter numerous challenges. Any interventions should always be carried out in a collegial manner with aim to support the site effort and mitigate their challenges.
The review of site performance is ultimately a team effort, where each study team (e.g., sponsor and CRO) brings available information about the site in question to the table and determines the course of action. Active monitoring methodologies that encompass the use of scientifically sound algorithms, clinical review, actionable intervention strategy, and team effort would improve and maintain accuracy and quality of data, which in turn, decrease placebo response.
Learn more about WCG and our solutions here or contact us here.
Source:
*Use of a novel study insight analytics (SIA) methodology to improve PANSS data quality and signal detection in a global clinical trial in schizophrenia. Schizophrenia Research, 267, (2024), 239-246.