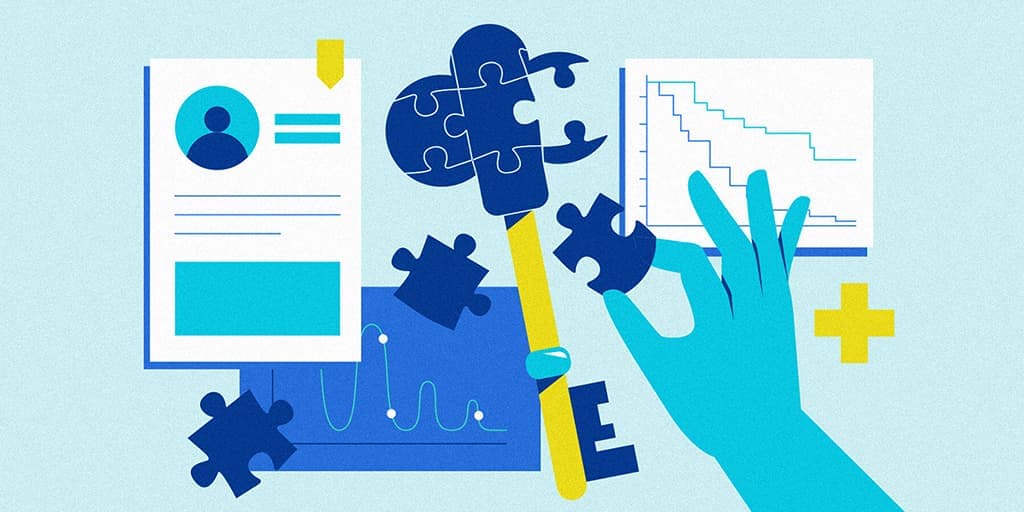
Data Quality 2.0: The Future of Real-World Evidence
The need for a data-quality framework is clear as real-world data (RWD) becomes increasingly complex and diverse. If we’re talking about EHR data quality though, let’s take a moment to dive a bit deeper into RWD from the EHR.
Flatiron Health’s RWD is curated from the EHRs of a nationwide network of academic and community cancer clinics. The richest clinical data, like stages of diagnosis and clinical endpoints, exists in unstructured fields. It’s challenging and complicated to pull that data, requiring both human interaction (including 2,000 human abstractors at Flatiron), machine learning, and natural language processing.
And it’s clear that quality matters. Recently, we’ve seen the growth of regulatory and policy guidance around its use by the FDA, EMA, NICE, Duke-Margolis Health Policy Center, and others. It’s also clear that quality is not just a single concept. It has multiple dimensions, which fall into the categories of relevance and reliability.
Assessing RWD: relevance.
Relevance of the source data has several subdimensions:
- Availability: Are critical data fields representing exposures, covariates, and outcomes available?
- Representativeness: Do patients in the dataset represent the population you want to study, e.g., patients on a particular cancer therapy.
- Sufficiency: Is the size of the population enough? Is there enough follow-up time in the data source to demonstrate the expected outcomes (e.g., survival, adverse events)?
These are traditionally assessed in support of a specific research question or use case. But at Flatiron, we must think more broadly to ensure our multi-purpose datasets capture variables that address the most common and important use cases (e.g., natural history, treatment patterns, safety and efficacy).
We also consider relevance as we expand our network – relying not only on community clinics that use our EHR software, OncoEMR®, but also intentionally partnering with academic centers that use other software. This enables us to improve the number of patients represented and make sure we’re aligned to where cancer patients actually receive care.
Assessing RWD: reliability.
Another dimension of quality is reliability. which has several critical sub-dimensions:
- Accuracy: How well does the data measure what it’s actually supposed to measure?
- Completeness: How much of the data is present or absent for the cohort studied?
- Provenance: What is the origin of a piece of data and how and why did it get to the present place? This includes a record of transformations from the point of collection to the final database.
- Timeliness: Does the data collected and curated have acceptable recency so that the period of coverage represents reality? Are documents refreshed in real time recency?
At Flatiron, we have developed important processes and infrastructure to ensure our data is reliable, with clear operational definitions. Our clinical and scientific experts help establish these processes, whether using an ML algorithm or guidance for human abstraction.
How does Flatiron ensure accuracy through validation?
We perform validation at multiple levels throughout the data lifecycle, e.g., at the field level at the time of data entry and at the cohort level. We use different quantitative and statistical approaches to validate the data at different levels – using a range of metrics depending upon the approach.
Figure 1:
Examples of validation approaches we use at Flatiron Health include:
- External reference standard: An example is National Death Index to validate a composite mortality variable, date of death (algorithmically derived from EHR and other sources such as the SSDI and obituary data). In Figure 2, we examined survival curves using our mortality variable to define the time to event outcome. We found that we got essentially the same curve using death dates from the NDI as from our mortality variable.
- Indirect benchmark: Derived from information from literature or clinical practice, e.g., we validated a novel real world progression variable by correlating to literature and related endpoints (Figure 2). Each curve represents a different Time-to-event (TTE) analysis, and you see expected correlations between progression free survival, overall survival, time-to-next treatment (TTNT) and time-to-progression (TTP).
- Internal reference standard: an approach we typically use when evaluating a novel curation process like machine learning. For example, in testing our ML algorithms, we used human-abstracted data as our “reference standard”. In Figure 2, you see two survival curves for patients with ROS1 positive NSCLC. The curves closely overlap, demonstrating very similar results with each curation process.
Figure 2:
Accuracy: verification checks.
Using clinical knowledge, we also monitor data and address discrepancies and outliers over time through different types of verification checks:
- Conformance is the compliance of data values with internal relational, formatting, or computational definitions or standards.
- Plausibility is the believability or truthfulness of data values.
- Consistency is the stability of a data value within a dataset, across linked datasets, or over time.
An example is using clinical expertise to evaluate temporal plausibility of a patient’s timeline of diagnosis, treatment sequences, and follow-up to assess whether data are logically believable.
Accuracy: completeness.
Completeness is a critical complement to accuracy to assess reliability. It’s not enough for data to be accurate, if it must be present first! We realize though that completeness in EHR based data is unlikely to be 100%.
To ensure completeness is meeting an acceptable level for quality, we place controls and processes in place across multiple levels. Data flows through many channels between the exam room and the final dataset – each step along the way is a point at which some elements may be lost, mislabeled, or inappropriately transformed.
We place controls and processes in place across multiple levels to monitor completeness. Thresholds are based on clinical expectations. In addition, integration of sources within or beyond the EHR can improve completeness.
In summary.
Understanding the quality of RWD is critical to developing the right analytic approach. But quality is not measured by a single number, it requires multiple dimensions. At Flatiron, applying cross-disciplinary expertise across the data lifecycle and a commitment to data transparency ensure our data users are equipped with the knowledge they need to generate impactful RWE.