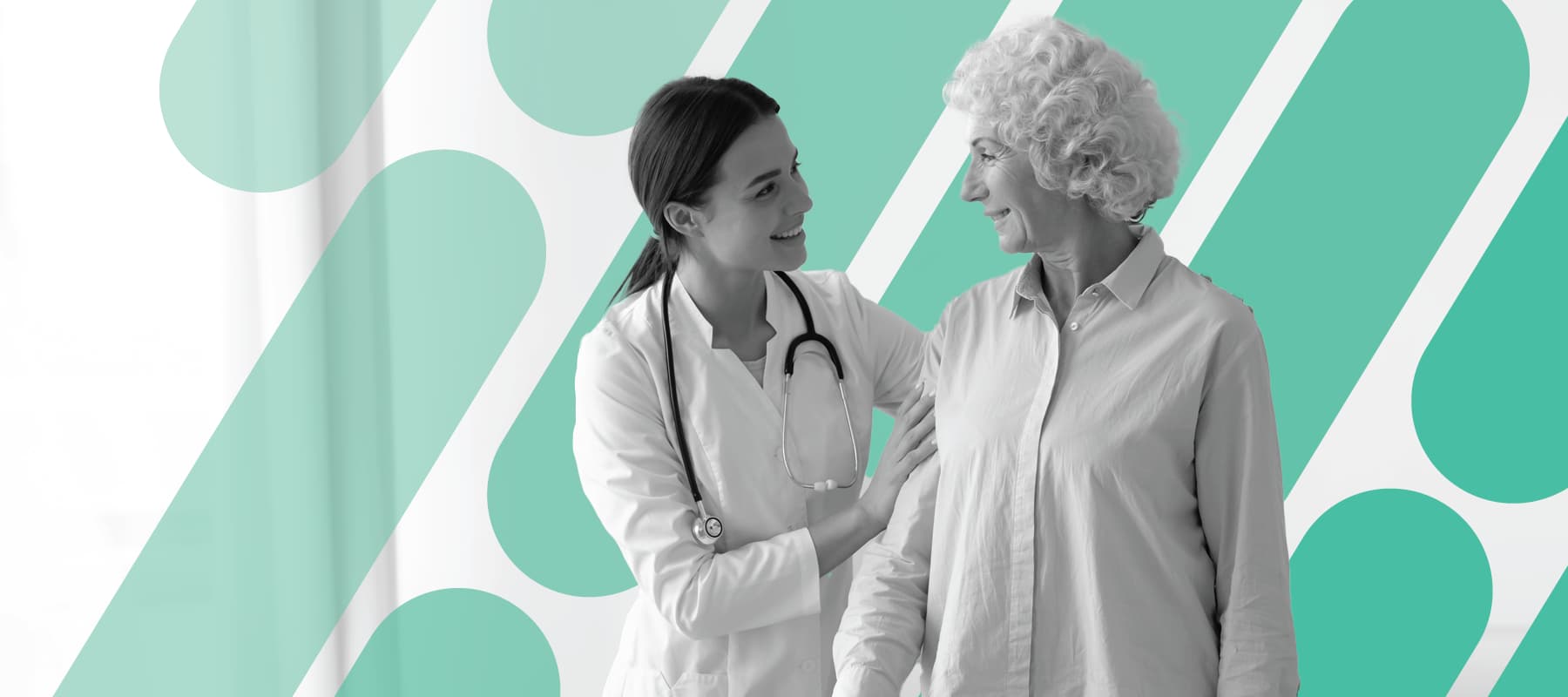
The role of AI in predictive biomarker patient matching
The emergence of personalized medicine is critical to cancer care as it promotes tailored treatments to patients to improve outcomes, and importantly limit exposure to non-efficacious treatments. Unlike the one-size-fits-all approach of traditional treatments, personalized medicines help ensure patients receive therapies that are most effective for their specific genetic makeup and disease pathology. The identification and application of biomarkers – molecules that indicate biological states – play a pivotal role in this process. Advances in the oncology space are forging ground in application of biomarkers for treatment decisions, and other therapeutic areas are swiftly following suit. Over the past decade response rates to treatment are 20% and increase to upward of 42% when a biomarker is used to select the appropriate treatment. Biomarkers can predict disease progression, response to treatment, and patient outcomes, thus enabling highly informed and personalized medical decisions across a broad array of therapeutic areas.
However, the lack of effective cancer treatment-specific biomarkers and disparate sources for biomarker data presents major hurdles for clinical uptake. While biomarkers can help guide clinicians in formulating the correct regimen at the individual patient level, the industry could benefit from unifying data storage as well as guidelines on application and use of biomarker data.
Biomarkers can be used across the continuum of hematology/oncology care, including risk assessment, screening, and differential diagnosis. Prognostic biomarkers can provide insight into the expected course of a disease, while predictive biomarkers provide insight into a patient’s anticipated response to a drug or intervention. Biomarkers can also play a role in therapeutic monitoring, providing insights into disease status, safety, and the efficacy of an ongoing treatment.
Challenges in biomarker patient matching
Despite its potential, biomarker-driven patient matching faces significant challenges. These include:
- Complex data integration: Biomarker data is often derived from and stored in diffuse sources, such as genomic sequencing, proteomics, reference laboratories and clinical trial databases. Integrating these multilayered datasets can be complex, time-consuming and is historically reviewed by a laborious manual process.
- Volume of data: The sheer volume of biomarker data generated is overwhelming and requires sophisticated tools to analyze and interpret it meaningfully.
- Heterogeneity of diseases: Diseases like cancer are highly heterogeneous, requiring nuanced understanding of individualized treatment strategies.
- Dynamic nature of biology: Biomarkers can change over time and vary among populations, making it difficult to develop static rules for patient matching.
Artificial intelligence, with its ability to process vast amounts of data and identify patterns beyond human capability, stands at the forefront of resolving a multitude of challenges. More specifically, machine learning (ML) algorithms — a subset of AI that enables systems to learn from data — are proving instrumental in biomarker patient matching.
Traditional biomarker discovery methods often focus on single quantifiable traits, limiting their ability to capture the intricate complexities of cancer biology. With AI, the concept of a biomarker evolves beyond a single measure to a generalized pattern. This approach allows researchers to analyze thousands of features and integrate complex data points into unified guidance. Applying stringent methodologies and good machine learning practices, such as preventing overfitting and data leakage, is essential to maintain the accuracy and reliability of AI-driven biomarkers.
WCG is actively engaged and pursuing AI in clinical research and precision medicine. Through a multidimensional approach, WCG leverages its deep domain expertise to develop state-of-the-art, AI-driven solutions. Consideration should be given to the following areas when looking for biomarker driven solution to patient matching:
- Data integration and management
Advanced AI techniques to integrate and harmonize data from multiple sources, streamlining the process of biomarker discovery and application. These sources include genomic data, electronic health records (EHRs), and clinical trial databases. By standardizing and integrating these data streams, the AI systems can achieve a holistic view of the patient’s biological makeup. - Sophisticated algorithm development
Application of bioinformatics experts to develop sophisticated ML algorithms specifically tailored to identify clinically relevant biomarkers. These algorithms should be trained on large, annotated datasets to ensure they can discern subtle patterns and interactions that may indicate a suitable biomarker for a given disease condition. - Predictive analytics
Predictive modeling is a key component of any approach. Using AI algorithms helps predict which patients are most likely to respond to specific treatments based on their unique biomarkers. This not only enhances treatment efficacy but also significantly reduces the trial-and-error often associated with traditional treatment methods. - Dynamic biomarker tracking
Understanding that biomarkers evolve over time, the use of AI for continuous monitoring and updating of biomarker data is imperative. This dynamic tracking allows for real-time adjustments in treatment plans, ensuring that patients receive the most relevant and effective therapies throughout their treatment journey. - Patient stratification
Patient stratification is another critical area where we have depth of expertise. AI algorithms develop profiles by grouping patients based on their biomarker data, helping clinicians to identify subgroups that are more likely to benefit from certain therapies. This can be particularly helpful in clinical trials to ensure the right participants are selected, thereby increasing the likelihood of trial success.
Ethical considerations and the future
However, with these advancements come ethical considerations. Issues around patient data privacy, transparency in how patient data is being applied and rigorous protection of patient interests. By promoting standards for the ethical use of AI in healthcare, the industry must aim to foster trust and ensure that technological advancements translate to tangible patient benefits.
The integration of AI in biomarker patient matching represents transformative leaps in precision medicine. With this technology, the possibilities for improved patient outcomes, greater clinical trial success, and reduction of healthcare costs are boundless.
To learn more about WCG and our solutions, reach out today. Unlock faster results with WCG.
Authors:
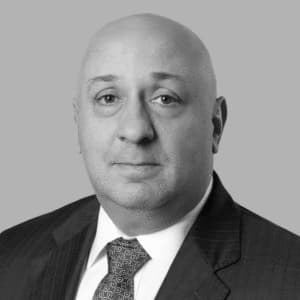
Senior Vice President,
Clinical Solutions
Mike Cioffi is a Senior Vice President with WCG in Clinical Solutions and provides unique insight and expertise to industry leaders in support of project delivery and implementing global enterprise solutions. Mike has worked in the pharmaceutical industry for more than 25 years and has extensive experience in various therapeutic areas with an emphasis on CNS indications and rare disease.
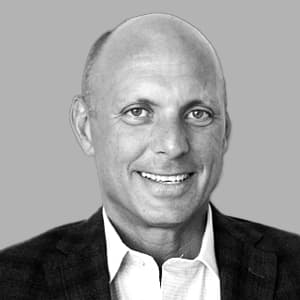
Senior Vice President,
Clinical Solutions
Brant Nicks is a Senior Vice President with WCG in Clinical Solutions and provides support and oversite for oncology trials. Brant has worked in clinical research for 28 years in various capacities with Biotechnology companies and Contract Research Organizations with an emphasis on Hematology/Oncology research.