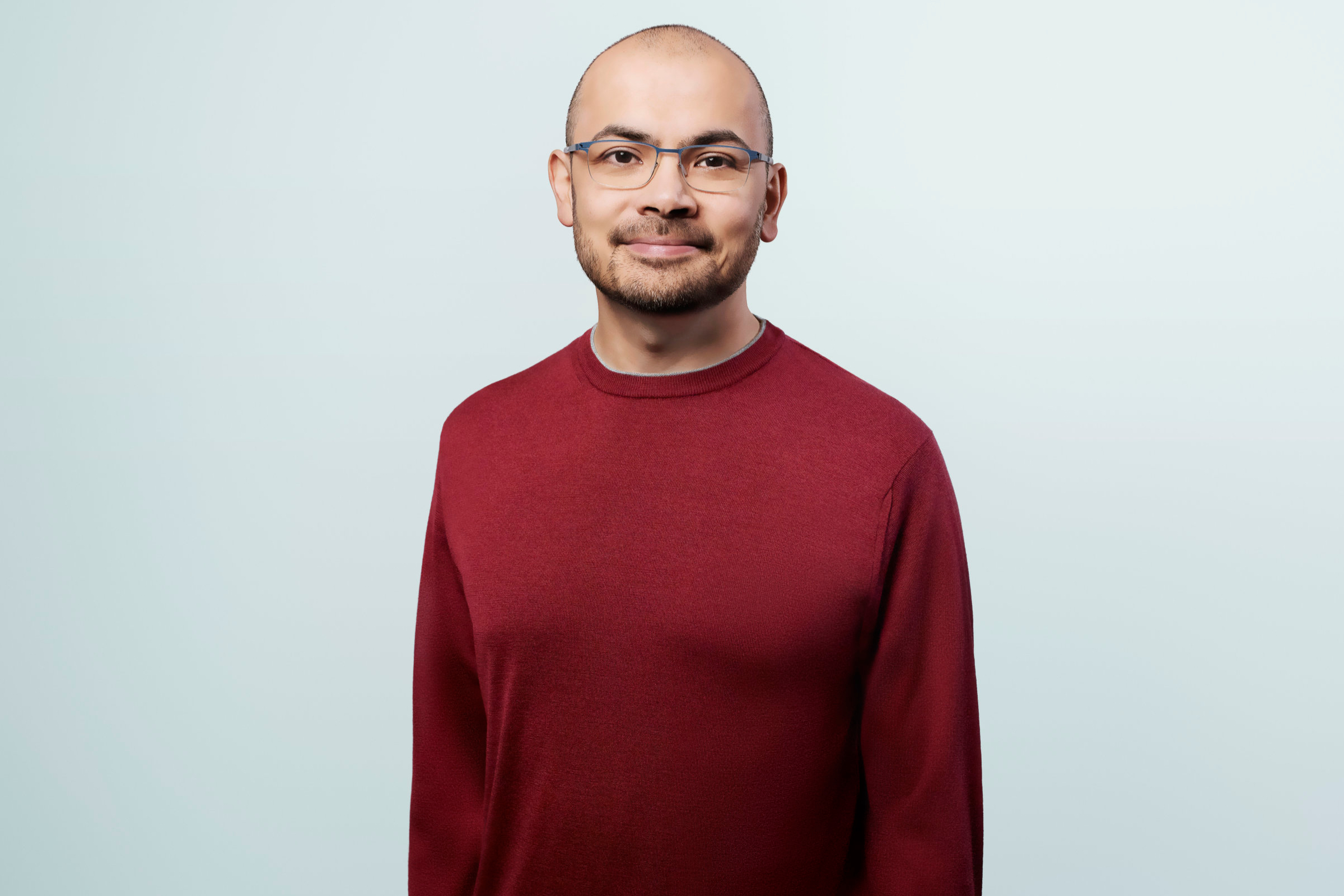
Nobel Prize winner Demis Hassabis talks about his vision for Isomorphic and AI’s future in biotech
In September, Endpoints News interviewed DeepMind’s Demis Hassabis. We’re republishing the interview today after Hassabis won the Nobel Prize in chemistry, shared with David Baker and John Jumper.
While Demis Hassabis is best known for steering Alphabet’s research efforts in artificial intelligence, the 47-year-old is simultaneously leading a secretive biotech startup looking to bring about an AI revolution in drug discovery.
Hassabis is the CEO of Google DeepMind as well as Isomorphic Labs, an Alphabet-backed company founded in 2021 to take DeepMind’s AI research in biology further into the drug industry. DeepMind has won a myriad of awards for AlphaFold2, its AI system that predicts protein structures and was published in 2021. Headquartered in London, Isomorphic has shared few details about what it’s working on in its roughly two years of existence.
In a rare, exclusive interview, Hassabis spoke with Endpoints News about his vision for Isomorphic and the future of AI in the life sciences. This is his first interview where he’s discussed Isomorphic, an Endpoints 11 recipient this year, in-depth. This conversation has been substantially edited and condensed for length and clarity.
Andrew Dunn: How’d Isomorphic Labs first get started?
Demis Hassabis: I’ve had in mind the idea AI could be used in a fundamental way for drug discovery for a very long time. I’d say it’s the number one thing I always wanted to apply AI to once it was sophisticated enough and powerful enough.
That’s obviously the big question: When is that moment the right moment? Throughout my startup and entrepreneurial career, I’ve learned timing is very important. Even if you have a great idea, if you’re 10 years too early, you’re in for a world of pain.
What I was waiting for was a signal that the timing was right. That, for me, was AlphaFold. I wanted that to work, not only to use as a foundational building block for what we’re doing at Isomorphic, but as a proof point that the AI methods were powerful enough, sophisticated enough that actually we could make a serious dent on this problem and reimagining drug discovery from first principles using computational methods, obviously including AI. So AlphaFold2 was the moment for me to press go on this.
Dunn: Before getting to the science, how is Isomorphic funded?
Hassabis: It’s an Alphabet company, officially, so it’s a sister company of DeepMind. The idea is a standalone company. It takes advantage of being part of the Alphabet family, mostly the vast amounts of compute we need if we’re going to achieve our mission. There’s close collaborations with DeepMind.
A lot of the culture things are similar to DeepMind. But it’s operating at a startup speed and energy and intensity. All our funding comes from Alphabet to begin with. That security allows us to go for the most ambitious version of the opportunity, which is to build this platform that will hopefully revolutionize the drug discovery process, as opposed to just go after one drug candidate or whatever.
Dunn: How much funding has Isomorphic received?
Hassabis: I don’t think it’s public information, so I can’t give you exact numbers. But it’s very well-funded for the stage that it’s at.
Dunn: You’ve described the goal as building more AlphaFolds, particularly in predicting how proteins interact with other proteins or small molecules, and in designing small molecules. What have you been able to do since getting started in November 2021?
Hassabis: I guess you’ve been following what I’ve said publicly quite carefully. It’s exactly that.
AlphaFold is only one small part of the whole drug discovery process. There’s all these adjacencies. We are focusing on small molecules first. We’re interested in biologics too, but we think small molecules is where we can make the most difference most quickly.
We’re very interested in the interaction space: protein-ligand interactions, protein-protein interactions, and the dynamic nature of biology. Also going into chemistry space, understanding chemical compound structures, how they might bind, binding affinities, these kinds of problems.
Just in that short sentence, I’ve outlined years worth of research work and many adjacent problems using advanced versions of AlphaFold as part of that mix. But we need many other things, things like predicting ADME properties, these types of things.
We need to make another half-dozen big breakthroughs.
Dunn: That reminds me of writing about the jump from the first version of AlphaFold to AlphaFold2. A lot of reworking from the ground up. How much of Isomorphic’s vision is based on extending AlphaFold versus building new models?
Hassabis: We’re always doing both actually. We’re always pushing existing methods to the limit. There’s usually more juice that can be squeezed. But we’re also investigating new ideas, new architectures.
We’re applying different techniques to these new domains. AlphaFold wouldn’t do things like ADME properties. That will be completely new technologies.
But with the interaction space, we’re working hard on that in fundamental ways with new versions of AlphaFold as well. There’s a ton of very interesting work going on, both at the DeepMind science team and Isomorphic. It’s complementary work. Isomorphic is more applied and more specific to drug discovery, whereas DeepMind is more fundamental research.
Dunn: How do you see Isomorphic provoking the drug industry’s status quo?
Hassabis: We’re posing some very interesting questions. One is how would drug discovery look if you did it from an AI-first perspective. Not as an add-on. My impression is, in traditional pharma, these are nice-to-haves. The chemists really do the work and then you double-check things in ADME simulations or whatever. We want to rethink that from the ground up.
That’s hard for Big Pharma to do because they’re entrenched in the ways of working they’ve been doing for decades. It requires a new approach, and that’s what we have.
If that’s true, can the drug discovery process do most of the research in silico and leave the wet lab stuff to validation? That’s the thesis, as opposed to doing the search for the compound experimentally, which goes much slower. My dream is we could reduce the time it takes by an order of magnitude, maybe, cost and time, and have a higher percentage success rate at the next stage if we’re correct and can build these half a dozen more AlphaFold-level breakthroughs.
Dunn: Are you solely focused on these fundamental breakthroughs? Are you building a pipeline, partnering with industry?
Hassabis: We’ll have more to say on this I think later in the year, but for now, what I can say is we’re open to both. We’re thinking about our own internal programs. We would pick targets and programs that we think are uniquely suited to our technology roadmap.
We’re open to partnerships with Big Pharma on interesting targets. I can’t tell you anything publicly right now.
Dunn: How do you summarize Big Pharma’s interest in working with Isomorphic? I see Big Pharma CEOs as generally smart people, who know AI is part of the future even if they don’t understand it. What have your interactions been like?
Hassabis: We’ve had tons of interest. Pretty much every Big Pharma company CSO has reached out to us at some point. We’ve had interesting discussions.
I think it’s bimodal: those that think it’s still far away and traditional methods will continue to be the way forward. Maybe AlphaFold is a one-off. They all use AlphaFold, by the way, and I think they found it useful, but they regard it more as a one-off tool versus those who think that maybe this is the future and would be interested to partner in some way. I’ve met both types.
Dunn: What’s your confidence level that AlphaFold is not a one-off, that this can be replicated and reproduced?
Hassabis: Over the years at DeepMind, I’ve become very good at trying to assess the difficulty of a problem versus the ideas we have, the talent we have, and I think it’s perfect timing. We’ve got the sweet spot just right. You want to be five years ahead of the pack, but not 50 years ahead.
Dunn: In the next three to five years, what does success look like for Isomorphic?
Hassabis: I would love us to have drug candidates in clinical trials. Also having an incredible platform and an engine that is producing dozens of these candidates on a very regular, short timeline basis. Our advanced candidate should be in clinical trials by then, so there’s actual, physical proof points that this is all working. And the platform has become pretty mature and very capable, maybe we’ve reduced the discovery time from years to months.
Dunn: Your background converges between AI and biology. Should more of the AI field focus on biology, or is there enough attention already?
Hassabis: I think more attention should be paid on these. And not just life sciences, but also AI for science in general.
But life sciences has always been one of my passions and the number one thing I always wanted to apply AI to, to help with curing diseases and understanding biology. In many ways, it’s the perfect regime. It’s very hard to imagine how you could mathematically describe a cell with a few equations.
Anyone can hand-wave and just go, “Apply AI to biology, it must work.” You probably hear that all the time with companies pitching you. But when you drill in, most of the time it’s not real. It also may just be, for that question, you should just use normal stats. There’s no need for AI, or AI can’t help with it.
That’s where the hype part comes in. Everyone just says they’re doing AI with bio, but in my opinion, very few people are, if you actually think about which teams are capable of building an AlphaFold from scratch. Obviously we gave it away, published it, but imagine we hadn’t done that and were doing it behind closed doors. What other groups could have built such a model? Very few, I think. More of that is needed.